About us
bAIome- the Center for Biomedical AI at the University Medical Center Hamburg-Eppendorf (UKE) was founded in 2019 with the mission to develop and translate Artificial Intelligence (AI) research into innovative solutions that integrate into clinical practice thereby improving and personalizing healthcare.
Team
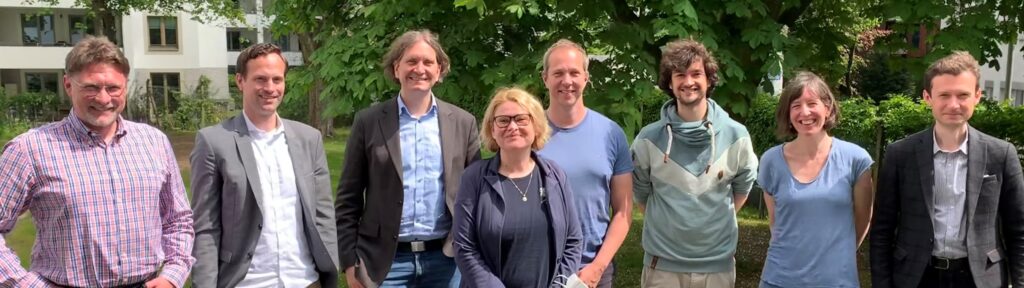
The bAIome team consists of UKE faculty members and researchers with long-standing experience in developing AI methods and their application in biomedicine.
Research
bAIome provides a nucleation point for biomedical AI research at UKE and creates unique opportunities for collaborative and interdisciplinary projects to develop novel AI approaches using clinical data. bAIome provides consulting and planning as well as performs research and implementation of AI projects with clinical relevance.
Selected Publications
Imaging & Image analytics |
---|
Werner, René, et al. “Clinical application of breathing-adapted 4D CT: image quality comparison to conventional 4D CT.” Strahlentherapie und Onkologie (2023): 1-6. Link |
Dietrich, Esther, et al. “Towards explainable end-to-end prostate cancer relapse prediction from H&E images combining self-attention multiple instance learning with a recurrent neural network” Machine Learning for Health, 38-53 Link |
Ristow, Inka et al. “Evaluation of magnetic resonance imaging-based radiomics characteristics for differentiation of benign and malignant peripheral nerve sheath tumors in neurofibromatosis type 1.” Neuro-oncology vol. 24,10 (2022): 1790-1798. doi:10.1093/neuonc/noac100 Link |
Schmitz, Rüdiger et al. “Artificial intelligence in GI endoscopy: stumbling blocks, gold standards and the role of endoscopy societies.” Gut vol. 71,3 (2022): 451-454. doi:10.1136/gutjnl-2020-323115 Link |
Genomics & Transcriptomics |
Khatri, Robin, and Stefan Bonn. “Uncertainty Estimation for Single-cell Label Transfer.” Conformal and Probabilistic Prediction with Applications. PMLR, 2022. Link |
Hausmann, Fabian, et al. “DiSCERN-Deep Single Cell Expression ReconstructioN for improved cell clustering and cell subtype and state detection (preprint).” (2022). Link |
Adlung, Lorenz et al. “Cell-to-cell variability in JAK2/STAT5 pathway components and cytoplasmic volumes defines survival threshold in erythroid progenitor cells.” Cell reports vol. 36,6 (2021): 109507. doi:10.1016/j.celrep.2021.109507 Link |
Adlung, Lorenz et al. “Machine learning in clinical decision making.” Med (New York, N.Y.) vol. 2,6 (2021): 642-665. doi:10.1016/j.medj.2021.04.006 Link |
Marouf, Mohamed, et al. “Realistic in silico generation and augmentation of single-cell RNA-seq data using generative adversarial networks” Nature communications 11 (1), (2020): 166 Link |
Menden, Kevin, et al. “Deep learning–based cell composition analysis from tissue expression profiles” Science advances 6 (30), (2022): eaba2619 Link |
Adlung, L. “Cell and Molecular Biology for Non-Biologists: A short introduction into key biological concepts” Springer Berlin Heidelberg 2022 Link |
Computational Neuroscience |
Panzeri, Stefano et al. “The structures and functions of correlations in neural population codes.” Nature reviews. Neuroscience vol. 23,9 (2022): 551-567. doi:10.1038/s41583-022-00606-4 Link |
Koren, Veronika et al. “Computational methods to study information processing in neural circuits.” Computational and structural biotechnology journal vol. 21 910-922. 11 Jan. 2023, doi:10.1016/j.csbj.2023.01.009 Link |
Kira, Shinichiro et al. “A distributed and efficient population code of mixed selectivity neurons for flexible navigation decisions.” Nature communications vol. 14,1 2121. 14 Apr. 2023, doi:10.1038/s41467-023-37804-2 Link |
Goulas, Alexandros et al. “Bio-instantiated recurrent neural networks: Integrating neurobiology-based network topology in artificial networks.” Neural networks : the official journal of the International Neural Network Society vol. 142 (2021): 608-618. doi:10.1016/j.neunet.2021.07.011 Link |
Fakhar, Kayson, and Claus C Hilgetag. “Systematic perturbation of an artificial neural network: A step towards quantifying causal contributions in the brain.” PLoS computational biology vol. 18,6 e1010250. 17 Jun. 2022, doi:10.1371/journal.pcbi.1010250 Link |
Damicelli, Fabrizio et al. “Brain connectivity meets reservoir computing.” PLoS computational biology vol. 18,11 e1010639. 16 Nov. 2022, doi:10.1371/journal.pcbi.1010639 Link |
Text Mining & Electronic Health Records |
Fuhlert, Patrick, et al. “Deep Learning-Based Discrete Calibrated Survival Prediction” IEEE International Conference on Digital Health (ICDH), (2022): 169-174 Link |
Multi-Type Data Integration |
Oller-Moreno, Sergio, et al. “Algorithmic advances in machine learning for single-cell expression analysis.” Current Opinion in Systems Biology 25 (2021): 27-33. Link |
Madan, Sumit, et al. “A semantic data integration methodology for translational neurodegenerative disease research.” (2018). Link |
Infrastructure
At the heart of bAIome is a state-of-the-art compute infrastructure for UKE students, researchers and clinicians to perform cutting edge AI in basic and applied biomedical research. These high-performance computing resources provide the necessary compute power and versatility to process large datasets and train complex models. Our team of skilled professionals offer help and support from introductory ‘when and how to get started with AI’ through to ‘advanced developer support’ by providing a functionally integrated infrastructure ecosystem.
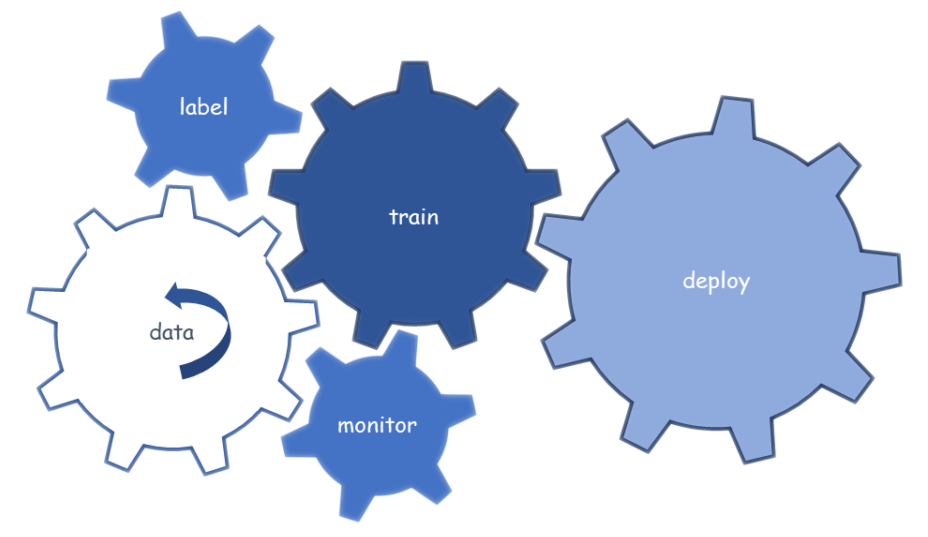
machine learning cycle requiring end-to-end support
Our compute services
At bAIome, we provide a number of infrastructure services to UKE employees including compute access, consultation & support, as well as training to support the development, training and running of AI/ML models. If you are wondering how to get started with processing your biomedical data or you are well on your way and simply need technical support, get in touch.
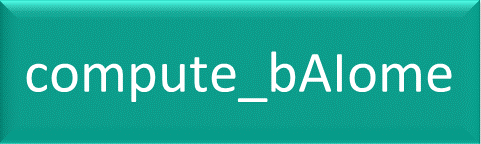
Access to our AI computing platform
- We offer access to our AI computing platform consisting of state-of-the-art computing hardware including NVIDIA H100 and NVIDIA DGX systems for all AI workloads.
- We provide a web service where you can develop and run your applications. e.g. via jupyterhub with several advantages including customizability, scalability, and suitability for various sized teams, and academic courses.
- We have storage services, such as object storage, for large projects.
- To best meet your needs, we have not one, but several production environments.
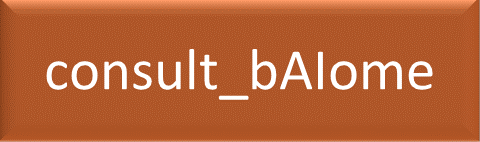
Our consultation experts can help guide you on when and how AI methods are best applied.
- We offer consulting before and during the development of a project.
- We provide technical support during the development phase and continuous during the lifetime of the project.
- We work to supply the best maintenance, monitoring, and optimization standards possible for every project.
- We offer best practice guides for all users.
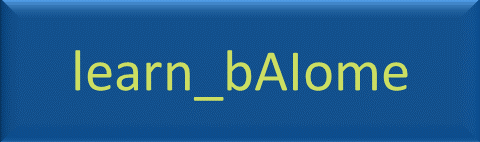
Training
- We provide technical introductory training on how to use our AI infrastructure
- We host regular live Q&A sessions for all infrastructure users
- For learning opportunities in the form of courses and workshops on diverse aspects of AI/ML, check out learn_bAIome main page
Teaching
bAIome offers a variety of seminars, courses, workshops, and one-on-one training for scientists, clinicians and students from various disciplines to become the next generation of AI experts in biomedicine.
seminar_bAIome and learn_bAIome provide learning opportunities in biomedical AI and data science with tailored formats based on the needs of students, clinicians, and researchers. We offer seminars, workshops and trainings within a structured framework which take into account background, programming skills and intensity to provide unique, focused, and effective courses.
AI courses in medical curriculum
Opportunities
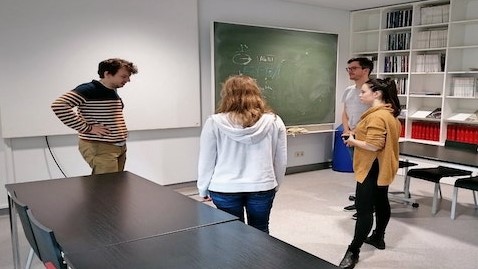
With state-of-the-art medical and computational facilities, resources, and talent, bAIome is an attractive place to learn, develop, and apply biomedical AI. The flexible exchange with other academic and industry partners provides the unique opportunity for students, clinicians, and scientists to contribute to translational research for healthcare applications.
Contact
for learning opportunities,
consulting & research, events
for infrastructure
Partners
One of bAIome’s most important roles is that of a hub between the forefront of AI research, clinical demand, and biomedical application. We view our collaborations with partners both in academia and in industry as essential for the innovative technological advancement of biomedical AI solutions. We are continuously expanding our network and are always ready to welcome new partners.
Stay In Touch